Artificial Intelligence (AI) is transforming industries at an unprecedented rate, enabling organizations to automate processes, predict trends, and personalize services. However, as AI becomes more embedded in our daily lives, ethical concerns regarding data storage and algorithmic fairness are coming to the forefront. Ensuring that AI systems are ethical and fair requires a deep understanding of how data is stored, managed, and utilized. This blog post delves into the role of data storage solutions in fostering ethical AI and ensuring fairness in algorithms.
Understanding Ethical AI and Fairness in Algorithms
Ethical AI refers to the design and deployment of AI systems that prioritize fairness, transparency, and accountability. These systems must be designed to avoid biases, respect user privacy, and operate in a manner that is aligned with societal values. Fairness in algorithms, on the other hand, involves creating models that make decisions without bias, ensuring equitable treatment across different demographics.
Bias in AI can arise from various sources, including biased training data, biased algorithmic design, and biased human oversight. Therefore, addressing these issues requires a holistic approach that encompasses data collection, storage, processing, and algorithm design.
The Role of Data in AI Ethics
Data is the lifeblood of AI systems. The quality, diversity, and management of data significantly influence the behavior of AI algorithms. Poor data practices can lead to biased models that reinforce existing inequalities, leading to unfair outcomes. Conversely, ethical data practices can help mitigate bias and promote fairness.
To ensure ethical AI, it is crucial to consider how data is stored and managed throughout its lifecycle. This includes data collection, storage, processing, and eventual disposal. Each stage presents unique challenges and opportunities for promoting fairness and reducing bias.
Challenges in Data Storage for Ethical AI
- Data Bias: One of the most significant challenges in ethical AI is data bias. Data used to train AI models often reflects historical biases present in society. For example, if a dataset used to train an AI model for hiring decisions contains biased data that reflects historical discrimination, the resulting model is likely to perpetuate these biases. Therefore, it is essential to ensure that data storage solutions support the collection and management of diverse and representative datasets.
- Data Privacy: Ethical AI must also prioritize data privacy. With the increasing amount of personal data being collected and stored, there is a growing need for data storage solutions that protect user privacy. This includes ensuring that data is stored securely, encrypted, and that access is restricted to authorized individuals. Additionally, data anonymization techniques can be employed to protect user identities while still allowing for meaningful analysis.
- Data Accessibility: For AI to be fair, it must be trained on diverse datasets that represent different demographics. However, access to high-quality, diverse datasets is often limited. Data storage solutions must therefore support the sharing and collaboration of data across different organizations and sectors while ensuring that data privacy and security are maintained.
- Data Governance: Effective data governance is crucial for ethical AI. This includes establishing policies and procedures for data collection, storage, and usage. Data storage solutions should support data governance frameworks that ensure data is collected and stored in compliance with legal and ethical standards. This includes ensuring data is accurate, up-to-date, and that individuals have control over their data.
Solutions for Ethical Data Storage
- Data Auditing and Monitoring: To ensure that data storage solutions support ethical AI, it is essential to implement data auditing and monitoring processes. Regular audits can help identify and address biases in the data, while monitoring can ensure that data is being used in compliance with ethical guidelines. Automated tools can be used to continuously monitor data usage and flag any potential ethical issues.
- Decentralized Data Storage: Decentralized data storage solutions, such as blockchain, can enhance transparency and accountability in data management. By storing data across a distributed network, decentralized solutions can reduce the risk of data breaches and ensure that data is managed in a transparent and secure manner. Additionally, decentralized storage can support data sovereignty, allowing individuals and organizations to maintain control over their data.
- Federated Learning: Federated learning is a technique that allows AI models to be trained on data from multiple sources without the data leaving its original location. This approach can help protect user privacy while still allowing for the development of robust and fair AI models. Federated learning can also support the inclusion of diverse datasets in AI training, promoting fairness in algorithms.
- Data Anonymization and Encryption: Data anonymization involves removing personally identifiable information from datasets to protect user privacy. Encryption, on the other hand, involves encoding data so that it can only be accessed by authorized individuals. Both techniques are essential for ensuring that data storage solutions support ethical AI. By anonymizing and encrypting data, organizations can protect user privacy while still enabling meaningful analysis.
- Ethical Data Curation: Ethical data curation involves the careful selection, organization, and management of data to ensure that it is representative, diverse, and free from bias. Data storage solutions should support ethical data curation practices by providing tools for data labeling, categorization, and validation. Additionally, organizations should establish guidelines for ethical data curation that prioritize fairness and inclusivity.
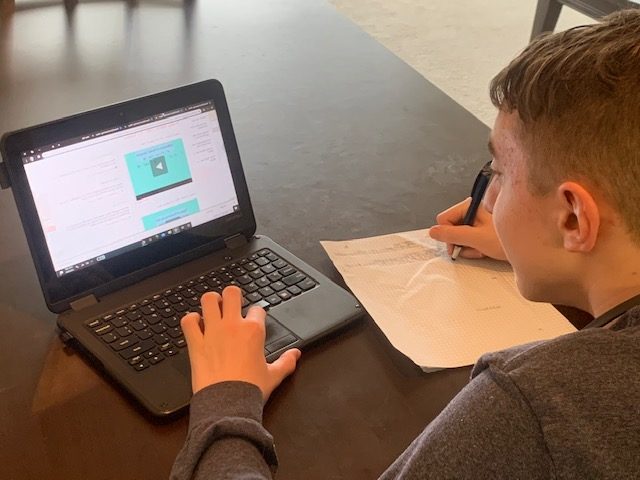
Promoting Fairness in Algorithms through Ethical Data Storage
Promoting fairness in algorithms requires a multifaceted approach that involves ethical data storage, algorithmic transparency, and ongoing evaluation. Ethical data storage plays a critical role in this process by ensuring that AI models are trained on high-quality, diverse datasets that are free from bias.
- Transparency and Explainability: To promote fairness, it is essential that AI models are transparent and explainable. This means that organizations should be able to explain how their models make decisions and identify any potential biases in the data or algorithms. Data storage solutions can support transparency by providing detailed documentation of data sources, processing methods, and usage.
- Bias Mitigation Techniques: Various techniques can be employed to mitigate bias in AI models, including re-sampling, re-weighting, and adversarial debiasing. These techniques rely on the availability of diverse and representative datasets, which can be supported by ethical data storage solutions. Additionally, organizations should regularly evaluate their models for bias and update them as necessary to ensure fairness.
- Inclusive Design: Inclusive design involves designing AI systems that consider the needs and perspectives of diverse users. This requires the inclusion of diverse datasets in AI training, which can be supported by ethical data storage solutions. Additionally, organizations should involve diverse stakeholders in the design and development of AI systems to ensure that they are fair and inclusive.
- Continuous Evaluation and Improvement: Ensuring fairness in algorithms is an ongoing process that requires continuous evaluation and improvement. Data storage solutions should support this process by providing tools for monitoring and evaluating AI models over time. Additionally, organizations should establish feedback mechanisms that allow users to report any unfair or biased outcomes.
Conclusion
Data storage solutions play a critical role in ensuring ethical AI and fairness in algorithms. By addressing challenges such as data bias, privacy, and accessibility, organizations can develop AI systems that are fair, transparent, and accountable. Ethical data storage practices, including data auditing, decentralized storage, federated learning, and data anonymization, can help promote fairness and reduce bias in AI models. For further help, tips, and advice about dropbox business server location, you may visit their page to learn more.
As AI continues to evolve, it is essential that organizations prioritize ethical data storage and management to ensure that AI systems operate in a manner that is aligned with societal values. By doing so, they can build trust with users, promote inclusivity, and contribute to the development of ethical and fair AI systems that benefit all.